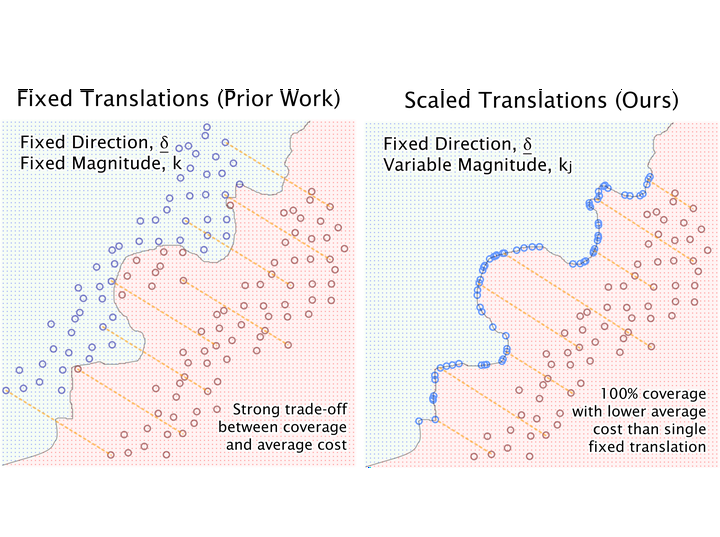
Counterfactual explanations (CEs) have been widely studied in explainability, though the major shortcoming associated with these methods, is their inability to provide explanations beyond the instance-level. While many works touch upon the notion of a global explanation, typically suggesting to aggregate masses of local explanations, few provide frameworks that are both reliable and computationally tractable. We take this opportunity to propose Global & Efficient Counterfactual Explanations (GLOBE-CE), a flexible framework that tackles the reliability and scalability issues associated with current state-of-the-art, particularly on higher dimensional datasets and in the presence of continuous features. Our framework permits GCEs to have variable magnitudes while preserving a fixed translation direction, to mitigate the commonly accepted trade-off between coverage and cost. Furthermore, we provide a unique mathematical analysis of categorical feature translations, utilising it in our method. Experimental evaluation demonstrates improved performance across multiple metrics (e.g., speed, reliability).
Published as a conference paper at ICML 2023.